Artificial Intelligence and Advanced Analytics in Real Estate
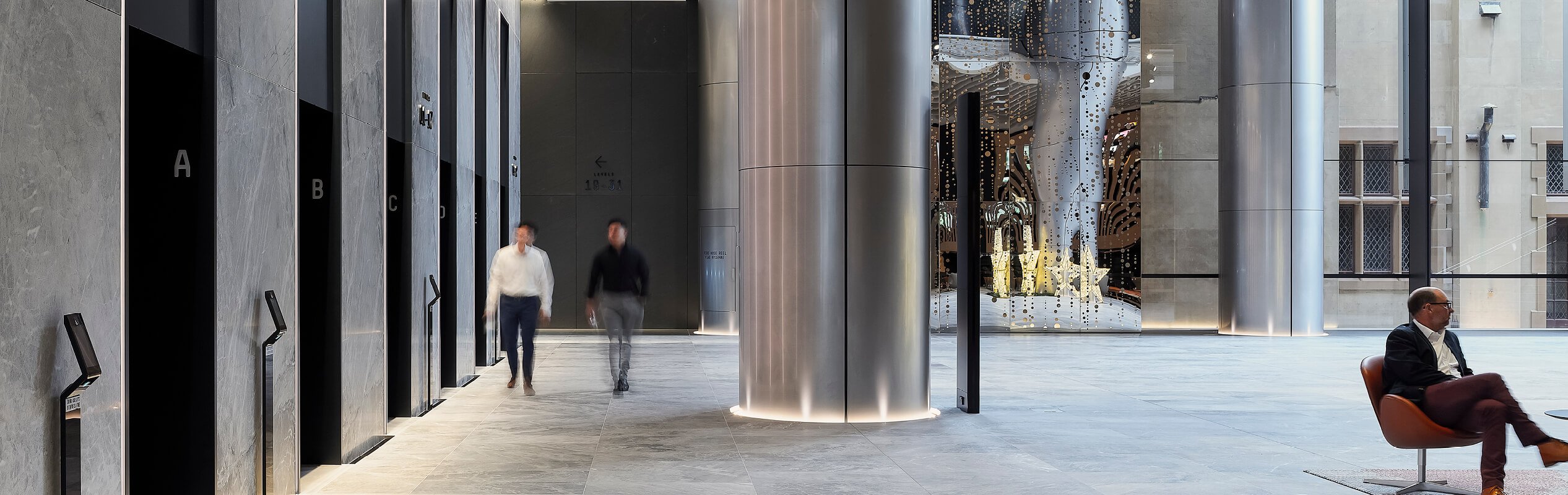
Contents
- Introduction
- What is Artificial Intelligence (AI)?
- What is Machine Learning (ML)?
- What is Deep Learning (DL)?
- What are the different types of artificial intelligence?
- What is the history of artificial intelligence?
- Why is artificial intelligence popular now?
- What are the benefits of artificial intelligence?
- What are the challenges with artificial intelligence?
- What are the risks of artificial intelligence?
Part 4: Applications and use cases
- What are the applications of artificial intelligence?
- Artificial Intelligence as a service (AIaaS)
- What is the future of artificial intelligence?
Download this paper as a PDF copy
Want to save this whitepaper for later?
Part 1: Foundations
1. Introduction
Big data, artificial intelligence, proptech and digital transformation get a lot of attention and rightly so. Data from Google shows that all of these terms have experienced significant growth over the past five years in particular.
Using just the lens of the property industry, it’s clear that many organisations in property are sitting on vast, ever-growing, quantities of data. But it’s not just about data.
In the next few years, data will become increasingly available. Leaders in the real estate industry will need to evolve quickly to unlock meaningful insights and thereby provide a better user experience for tenants, investors and customers – or risk being left behind.
At Investa, we are actively investing in data and decision intelligence. We believe that it is first about asking the right questions, then having the right people, data and technology to find the answers. Investa believes that organisations will derive a real competitive advantage by building decision intelligence capability, and that the opportunity is “now.”
Forward-thinking property companies will progressively be able to see and realise value that the general property market cannot see. As our models and data sets become more sophisticated over time, we will see increasing levels of return on the investment in this capability.
Part 1 of this article defines AI, Machine Learning (ML) and Deep Learning (DL) and looks back at its history, how we got here and how we can benefit.
Part 2 looks at six force multipliers that businesses use to drive a ROI from data and AI.
Part 3 focuses on some of the challenges and risks that come with AI.
Part 4 outlines some specific real estate use cases of AI.
2. What is Artificial Intelligence (AI)?
Artificial intelligence refers to the wide-ranging simulation of human intelligence by machines.
The goal of AI is to solve the kind of problems or perform the types of tasks that are usually completed by humans, with our natural intelligence.
There are two main types of AI: The first is “Weak AI” or Artificial Narrow Intelligence; and the second is “Strong AI” or Artificial General Intelligence.
AI will typically demonstrate some of the following characteristics: planning, learning, reasoning, problem solving, perception, motion, manipulation and to a lesser extent, social intelligence and creativity.
According toArtificial Intelligence: A Modern Approach, by Stuart Russell and Peter Norvig, AI can be defined by four fundamental approaches:
1. Thinking humanly
2. Thinking rationally
3. Acting humanly
4. Acting rationally
The first two approaches centre around thought processes and reasoning, the latter two focus on behaviour.
3. What is Machine Learning (ML)?
Understanding the difference between artificial intelligence, machine learning, and deep learning can be confusing.
Venture capitalist Frank Chen made this distinction:
"AI is a set of algorithms and intelligence to try to mimic human intelligence. Machine learning is one of them, and deep learning is one of those machine learning techniques."
ML feeds a machine (computer) data and uses statistical techniques to help it learn how to progressively get better at a specific task. Machine learning consists of both supervised learning and unsupervised learning.
4. What is Deep Learning (DL)?
Deep Learning (DL) is a type of machine learning that runs data through brain-inspired neural network architecture. The neural networks allow the machine to go deep in its knowledge-base, making connections and weighting data.
5. What are the different types of artificial intelligence?
Artificial Intelligence falls typically under two categories: Narrow (or weak) AI and artificial general intelligence (AGI) or strong AI.
a) Narrow (or weak) AI
Narrow or weak AI operates within certain limitations and is a simulation of human intelligence. It's often focussed on executing a single (or narrow) task really well.
Much of narrow AI is powered by machine learning and deep learning. This AI can often outperform humans in a specific niche task — especially if the task relies on historical knowledge and data.
Some examples of narrow AI include technology that is broadly used: Google, Siri, Alexa, self-driving cars, chatbots, translators, social network recommendations. The most famous example here is IBM's Watson.
At Investa, we have been using AI/ML in our business for years. For example, we use cybersecurity AI software to detect potential threats, and search engine AI across our internal files and emails.
In addition to these baseline use cases, we are focused on narrow AI use cases that make an impact on our business, customers, investors and employees.
For example, identifying new development sites to acquire, or what tenants are the best fit to occupy our buildings. This application of narrow AI allows us to augment what we do as humans and remove some of the repetitive tasks in our business.
b) Artificial General Intelligence (AGI) or strong AI
Artificial general intelligence is the creation of a machine with human-like intelligence that can be applied to any task. It seeks to replicate the cognitive abilities of the human brain.
When presented with an unfamiliar task, a strong AI system should be able to apply knowledge from another domain or task to find a solution autonomously.
Strong AI systems tend to be more complicated, and is often the subject ofscience fiction. We are not yet deploying AGI into our businesses, but it is definitely on our radar for the future.
6. What is the history of artificial intelligence?
Artificial intelligence had its start in antiquity by mathematicians and Greek philosophers. But when we think of artificial intelligence in modern-day terms, its history spans less than a century. Here are some of the key milestones in AI.
1943 –Warren McCullough and Walter Pitts publishA Logical Calculus of Ideas Immanent in Nervous Activity.The paper proposed the first mathematic model for building an artificial neural network.
1952 –Arthur Samuel wrote the first game-playing program for checkers with sufficient skill to challenge a human player.
1958 –John McCarthy develops the AI programming language Lisp and publishes the paperPrograms with Common Sense.The paper proposed a complete AI system with the ability to learn from experience as effectively as humans do.
1981 –Digital Equipment Corporation develops R1 (also known as XCON), the first successful commercial expert system. Designed to configure orders for new computer systems, R1 kicks off an investment boom in expert systems that will last for much of the decade, effectively ending the first “AI Winter”/ R1 saves the company $40 million a year by 1986.
1997 –IBM’s Deep Blue chess machine beats world chess champion Gary Kasparov.
2002 –iRobot creates Roomba, the first commercially successful robot for the home. It autonomously vacuums the floor while navigating and avoiding obstacles.
2008 –Google launches an app on the new Apple iphone with speech recognition.
2014 –Google makes the first self-driving car to pass a state driving test.
2016 –Google DeepMind’s AlphaGo defeats world champion Go player Lee Sedol. The complexity of the
ancient Chinese game was seen as a significant hurdle to clear in AI.
2018 –Alibaba developed an artificial intelligence model that scored better than humans in a Stanford University reading and comprehension test, scoring 82.44 on a set of 100,000 questions.
7. Why is AI popular now?
Increased computer power has been a significant driver for AI, especially infrastructure speed, availability and scale. What used to be run in specialised labs with access to supercomputers, can now be deployed on the cloud at a fraction of the cost.
Thanks to critical mass and the awareness of natural language personal assistants, like Siri and Alexa, AI has also become mainstream. Large players are investing heavily in it and AI has fuelled an explosion of AI-based start-ups across industries such as healthcare, finance, insurance, real estate, commercial real estate and many more.
Big data is another driver for AI in recent times. The increase in data has created a strong demand for solutions that go beyond simple data analysis and instead promise deep, new insights and intelligence. Data is the fuel for AI, and as data sets become more accessible, higher quality and cost less, the barrier to entry for building effective AI models decreases.
As real estate is the largest asset class in the world, there is a large amount of data that we can use for our use cases at Investa. This data is becoming better, but is still disparate, incomplete, hard to access and difficult to structure.
Investa partners with data providers such asEmpirical CREto build robust data sets, but there is a large amount of work, creativity and knowledge required to hunt and structure the data we need.
“Investa understands the benefits that can be derived by leveraging both the intelligence in our data, as well as in our people, to ultimately drive business value.” Jonathan Callaghan, CEO, Investa.
“Before Empirical CRE entered the market (commercial real estate), data providers focused on limited groups of properties using the basket approach, which in turn provided a limited picture of certain datasets without access to real-time or frequent captures of trends. Empirical CRE's objective is to track all properties regardless of size, geography, or type across all major metro areas to provide landlords, agencies, and their research teams a platform with standardised content to aid critical decision making in one of the highest value real estate markets in the world,” said Doug Curry, EmpIrical CRE.
As the data in real estate matures, increasing numbers of property companies will be able to access and derive value from these data sets, which will allow them to leverage AI/ML even further.
8. What are the benefits of AI?
Humans and AI work like a partnership.
AI excels at complexity and finding patterns in vast quantities of data – which would be impossible for a human to compute. Humans excel at creativity and ambiguity — using the insights from AI to support decision making and negotiation.
Investa has partnered with cutting-edge AI platform provider SparkBeyond, to accelerate and amplify the way in which the business collects, organises, analyses and draws insights from data.
"Using AI to generate data-driven insights to inform decision intelligence is a cultural shift for many organisations. In our experience, doing it well requires strong collaboration across the organisation to allow AI and humans to play to their strengths. It has been a pleasure to work closely with the Investa team to support them through this cultural shift and we're excited to see this translate into real business value.", Katherine Leong, Impact Strategist, SparkBeyond.
The benefits of AI complement rather than replace humans. Here are some of the benefits of AI.
a) Reduced “human error”
Humans as we know aren't perfect and we make errors.
Using AI, computers can avoid (or reduce) making these "human errors," providing greater accuracy and precision. This has the effect of increasing productivity in the economy and driving growth.
b) Less risk
Reducing the risk to human life is one of the most significant advantages of AI.
Robots driven by AI can be used on Mars, to defuse a bomb and even for natural disasters.
From a business perspective, AI can remove risks by flagging anomalies like fraudulent transactions, it can monitor equipment and it can even suggest preventative maintenance.
c) 24/7
Another benefit of AI is the sheer workload it can carry.
Where the average human can work for 8 hours a day, including some breaks, AI machines can be operational 24/7 and without any downtime.
For the property industry, there is a benefit for use cases that require constant monitoring — like optimising energy usage in a building for example.
d) Repetitive jobs
As part of life, humans perform many repetitive jobs. Think of manually inputting data into Excel spreadsheets, replying to emails or verifying documents.
With deep learning and machine learning, AI can “become smarter over time.” It can take care of repetitive tasks and increase business efficiency.
In the property industry, AI can be used to analyse tenant requests and anticipate what types of requests will be logged (and when). This allows organisations to better predict tenant issues and enables them to provide better, proactive customer service.
e) Digital assistants
In the realm of sales, marketing and customer service, AI can be used to augment human interaction.
For example, chatbots can be used on a website and social media to engage with users and pre-qualify them with questions that can better direct their enquiry within the business. Chatbots can also operate 24/7, allowing users in different time zones to chat with a business at a time that suits them.
At Investa, we are starting to experiment with digital concierge, which can provide service at our buildings outside of standard business hours..
f) Faster decision making
Thanks to the sheer amount of data points that AI can process and analyse, AI can enable faster decision making.
For example in September 2019, Investa partnered with AI-platform provider,SparkBeyond.
The purpose of the partnership was to accelerate and amplify how Investa collects, organises, analyses and draws insights from data, at a scale not previously possible.
AI enables Investa to harness previously untapped insights from hundreds of internal, external and open-source data points, enabling unprecedented decision intelligence across a range of core business areas including acquisitions, tenant attraction and retention, pre-emptive building operations, optimal deployment of capital and aligning investors with the right opportunity at scale.
1. Force multipliers and decision intelligence
Data, AI/ML and insights are force multipliers. They are tools to solve business problems, and they allow organisations to leverage people, systems and resources to maximise impact.
For example, Google is a force multiplier that amplifies the information historically held in books and articles.
It’s much easier to type a keyword into a search bar, than it is to physically sift through the library stacks for information.
“At Investa, we are multiplying and amplifying the talents, experience and creativity of our people through data & AI.”
Joanna Marsh, General Manager, Innovation & Advanced Analytics, Investa
2. Six force multipliers of Decision Intelligence
a) Explore
When we first talk about data, insights and artificial intelligence, it can be tempting to see AI as something that will solve all of our business problems.
And while AI can make a massive impact on any business, the key to successfully growing an analytics and insight capability is to focus and prioritise.
The first step is to explore which business problems — if solved — would help execute the business’ strategy.
Practically, this can look like a workshop, with key stakeholders across the business, where we discuss the business problems and the impact that solving these problems would have on our strategy and business performance.
An output of this workshop may be a long list of [50+] use cases that would make a difference.
For example, in commercial property, one potential problem could be difficulty in accessing the required volume or type of properties or development sites to achieve growth.
So when thinking about that problem in this explore phase, we would generate a use case or potential solution to that problem.
At Investa, one solution for us, was creating an AI-powered decision intelligence model that allows us to find and evaluate off-market opportunities so that we can broaden our asset and development pipeline.
b) Evaluate
The next phase is to prioritise and evaluate these use cases against the business strategy and also against the feasibility of successfully being able to execute.
Most AI use cases are evaluated against a simple 2 x 2 matrix of impact vs. feasibility. From our experience, we have found that this approach can be insufficient and can lead to issues downstream.
More detail is required to adequately understand the potential benefits and also the risks of embarking on each use case.
At Investa, we use decision thinking in partnership with Catalyze Consulting — a company that enables structured decision making.
In collaboration with Catalyze, we have built a Multicriteria Decision Analysis (MCDA) tool to support our decision making and to evaluate our decision intelligence use cases.
In addition to financial return, we have criteria that underpin the four pillars of Investa’s business strategy.
We also consider impact over time, fit to data, probability of success and time to return. This sophisticated model allows us to prioritise which use cases to build first and helps us communicate to stakeholders. It is also essential to talk about the conditions for launch (e.g. alignment to business' strategy, senior sponsors, funding, and resources to deliver the desired outcomes).
“Applying Decision Thinking to Data, AI/ML & Insights use cases gives senior executives the confidence and clarity they need to make decisions on where to focus attention to win. A properly structured decision process enables clear comparison between options, even when there is disparate data and information sources, and provides a clear picture of the value (and value for money) each option would deliver against the things the leadership really care about.
Most importantly, the creation of bespoke decision criteria and adoption of a rigorous decision method mean that organisations can deliberately gear their decisions toward innovation, capability gaps or any other area they want to go after, while understanding the value trade-offs between options,” said Paul Gordon, CEO Catalyze.
Below is a tornado chart showing how we prioritise data and analytics use cases against the cumulative weighted value of each. This allows us to clearly see which problems if solved, will deliver the largest ROI against our strategic criteria.
c) Experiment
The third phase involves empowering your teams to be creative with the data.
In the property industry, there isn't much precedent around data and AI — it’s basically pure innovation. Here at Investa, we’re building solutions in an agile, creative manner — not following a well-tested roadmap.
This means that teams have to experiment with data. Data is the lifeblood of AI and the data typically available in the property industry is often sparse, incomplete, expensive or non-existent. Businesses in these types of industries need to think differently.
At Investa, we are partnering with data providers to co-create data sets to benefit both parties. We are building our own structured data sets from external and public data and feeding those into our models. Often we find a signal in data that initially appears to have no value. It is about finding creative solutions and continually following the thread. We are always data hunting.
“We are delighted that our platform has been able to deliver such impressive results to Investa, who have shown great foresight to develop a strong, data-driven culture internally. AI-driven decision intelligence is empowering Investa with strong and actionable insights for sustainable growth and measurable impact”, Amir Haramaty, Chief Commercial Officer, SparkBeyond.
d) Engage
Combining artificial and human intelligence to validate, learn and engage with the data is an essential part of phase four.
Humans are vital in the AI-feedback loop and by using human intelligence, we can help AI models to learn, but more importantly, we can build products that deliver on the impact promised to the business.
At Investa, we have built an AI-powered decision intelligence tool which evaluates and monitors all properties in a given market that match our investment criteria.
We also include our capital transactions team in the AI-feedback loop and collectively assess the results of the modelling.
The data then provides guidance us to why specific target properties are a "yes" or a "no" and tell us where to look next — be that new asset classes, new geographical markets or something else.
While AI is exceptional at completing large amounts of the "janitorial" tasks of working through and cleaning the data, humans excel at the nuances and creativity of the deal negotiation – together, the impact is realised.
To set up AI for success in an organisation, it really is imperative to have the support of the C-suite and the senior leadership team.
At Investa, innovation, data and AI is championed by our CEO and CIO, and the entire executive team is behind the effort.
“Innovation is a key strategic pillar of our strategy. We are prioritising investments in innovation projects and ventures, focused on leveraging new technology and data,” said Peter Menegazzo, Chief Investment Officer.
“We have invested heavily in growing an innovation culture within the business, encouraging our people to look at problems and opportunities differently, to be data driven, to question things and trial different ideas, in order to reach the optimal, customer-centric outcomes.”
This support gives much-needed air to the effort of building models and provides the impetus for stakeholders across the business to engage.
Without the engagement of the business and senior leadership, the risk is that AI and data gets siloed and has less impact.
e) Execute
Once a new innovation product has been placed into the business (into the wild), it is vital to support people to leverage the tools and engage. This is where we realise the ROI of the use case and where the business benefits.
At Investa, we are very conscious of supporting our people in this journey.
An example of how we embed knowledge and learnings across the business is the creation of our company-wide skills training program, the Innovation Institute.
Now in its second year, the initial Innovation Institute program focused on educating participants on the Design Thinking innovation process. This year’s curriculum has moved to a focus on Data Literacy. As part of this, we are educating our people in all things data, insights and AI / ML.
“The Innovation Institute is an industry leading initiative that provides participants with the opportunity to gain unique and tailored insights that align with the changing nature of their roles and allows Investa to deliver superior client outcomes while achieving the organisation’s strategic goals.
“We have flexed our creative muscles to come up with a highly agile course for 2020 that caters to all levels, ensuring that Investa’s employees will be at the forefront when it comes to application of core concepts and adoption of new technologies,”
– Lorna Nolan, National Marketing & Communications Manager.
f) Expand
Once a use case has been completed, it can be tempting to move on to the next business problem, however it’s vital to manage the ongoing optimisation and use of the models in the business in order to yield maximum value.
At Investa, we focus on continuous improvement of our models, incorporating new data sets, sharpening the tools and making sure our people are still getting value. From there, we leverage that work into the next set of use cases, so we are continually evolving our tools.
“Investa is at a pivotal point in its journey. We’re looking at all core business activities through an innovation and technology lens,” said Emily Lee-Waldao, Group Executive, Brand & Innovation.
“Our internal and external investment in innovation projects and ventures is focused on leveraging new technology and data to do our core business better, improve the operation and performance of our buildings and expand our capability to grow in areas that are aligned with the strategic direction of the business.
Part 3: Challenges and risks
1. What are the challenges with artificial intelligence?
a) Demonstrating a clear return on investment
One of the main challenges of artificial intelligence is not primarily technical, but rather how to accurately predict, and then achieve ROI from the investment in AI.
According to a 2019 MIT Sloan Management Review and Boston Consulting Group (BCG) report, "seven out of 10 companies surveyed report minimal or no impact from AI so far. Among the 90% of companies that have made some investment in AI, fewer than 2 out of 5 report business gains from AI in the past three years. This number improves to 3 out of 5 when we include companies that have made significant investments in AI. Even so, this means 40% of organisations making significant investments in AI do not report business gains from AI."
According to a report by Deloitte in 2018 on "State of Enterprise AI" — the top three challenges with AI were implementation issues, integrating AI into the company's roles and functions and data issues.
In a 2018 McKinsey Global Survey of AI, "most respondents whose companies have deployed AI in a specific function report achieving moderate or significant value from that use, but only 21 percent of respondents report embedding AI into multiple business units or functions."
At Investa, we have recognised this challenge and have addressed it through effective prioritisation of our use cases, clearly identifying baseline statistics such as time spent and cost, so we can effectively estimate the ROI of each use case.
We then measure that ROI as we go and again once the product has been in use within the business. This is an ongoing process and we keep this front of mind as a standing agenda item at our quarterly Innovation Investment Committee meetings.
“The final objective is always to link back to business strategy. Advanced analytics and insights are only as valuable as the business outcomes they drive,” said Joanna Marsh, General Manager, Innovation & Advanced Analytics, Investa
b) Scarcity of technical skills
Given that AI is still an emerging technology, there is a limited amount of people who have the required technical and business acumen to work with it.
Many small to medium sized businesses don’t have the budgets available to hire in-house Data Scientists or other specialist roles required to work on the AI.
At Investa, we believe strongly in the power of partnerships to access world-class talent. Through our partnership with SparkBeyond, we have created a team of experts with niche skills such as natural language processing that we can apply to our use cases.
“In a time of great transformation, many companies are struggling to innovate in a way that drives real business impact. Being able to see patterns, derive insights and solve business problems through artificial intelligence and machine learning, allows us to make better decisions.
As we move into the future, we will be able to leverage these insights to uncover opportunities that are not yet articulated clearly or adequately serviced in our industry,” said Joanna Marsh, General Manager, Innovation & Advanced Analytics, Investa.
"Utilising AI platforms like SparkBeyond enables small teams of industry specialists to outsource the labour-intensive tasks of data processing, formatting and analysis, to the AL/ML platform. This enables the Investa team to spend more time focusing on the findings to create innovative, new ways to solve key business problems." Will Cosby, Senior Data Scientist
c) Data quality and quantity
Data is the lifeblood of AI. The quality of any platform, algorithm or insights relies on the data that it's using, and AI needs a considerable amount of data to learn and identify meaningful patterns. The better the data, the better the applications of AI and machine learning.
In the property industry, we are working in a data-constrained environment. We have access to niche data sets in each market, but these data sets vary enormously between different markets.
For example, we have partnered with Empirical CRE, a data provider that entered the Australian market two years ago. Empirical have built a detailed data set for office and industrial properties across different geographical markets.
This data set is key to many of our use cases because it includes data about tenants, rents, lease expiry dates and building profiles — as well as sales history. This level of data is extremely useful, but in other markets there are challenges in obtaining data with the same level of granularity. As a result, we are having to create our own data sets through a variety of mining techniques.
“The property industry is coming up the data maturity curve fast, but we are still spending the majority of our time collecting, cleaning and most importantly, analysing different data assets to understand its value. This is where thinking creatively about the problem and its relationship to what the data is telling us is imperative in delivering a unique solution to drive competitive advantage.
Developing a high volume, varied and incongruous foundation of data that can be blended and ingested into our AI/ML modelling is the secret sauce. In true innovation style, the data asset development never ends, as each use case presents a set of challenges that can often be solved with better information .” Billy O’Neill, Digital Manager, Data & Insights
d) Integration
Fully integrating AI into your organisation, is a lot more complicated than adding a plugin to your website or an extension to your browser. The entire interface and workflow have to be setup to reflect your unique business goals.
Data infrastructure, data storage, data labelling, testing and a feedback loop also need to be considered as part of the integration.
To do this, a strong partnership with your Chief Information Officer (CIO) is vital. At Investa, we have tightly aligned our information technology team and our data, innovation and analytics team, so that we can innovate and really create value.
2. What are the risks of artificial intelligence?
The previously mentioned Deloitte in 2018 on "State of Enterprise AI" report identified the following top seven risks of AI according to executives.
a) Cybersecurity
In the Deloitte report, 23 percent of executives ranked "cybersecurity vulnerabilities" as their top AI concern or risk. Researchers have found that machine learning models can have difficulty detecting adversarial input — data intended to deceive the model.
b) Legal responsibilities
All types of systems and technology present a range of legal and regulatory risks, including AI.
Companies looking to assess legal risk from the implementation of AI need to take a holistic approach to liability, assessing their risk at multiple levels: liability for the intention and effect of an AI system, liability for the performance of the algorithm, and liability arising from the data used to train the algorithm.
c) Failure
Thirty-nine percent of respondents in Deloitte's report, cited the failure of an AI system in a mission-critical or life-or-death situation. Placing strategic decisions or mission-critical actions entirely in the hands of an AI system would certainly entail unique risks.
For example, the use of AI in autonomous vehicles has been implicated in several accidents, some fatal.
d) Regulatory non-compliance
In a post-General Data Protection Regulation (GDPR) world, privacy and explainability has never been more important.
The GDPR which has come into force in Europe, sets specific rules around privacy. It also mandates that companies who use personal data to make automated decisions affecting people must be able to explain the logic behind the decision-making process.
These privacy and regulatory concerns, can add an extra layer of risk for companies looking to invest in AI.
e) Reputation
Reputational risk is another factor for organisations to consider. For example, data leakages and “getting hacked” are real probabilities.
Organisations face a risk to their reputation and brand, when their intellectual property, assumptions or insights are discriminatory for example.
f) Ethics
When it comes to AI, ethics is a top-of-mind factor to consider.
From spreading "fake news" to misusing personal data and biased algorithms, AI can pose a range of risks that are less tangible but no less critical.
At Investa, we believe strongly in AI as a “Glass Box” where we can see, investigate, and interrogate the assumptions of the AI model.
We also continually monitor our AI program for data drift.
Our AI modelling might be set up ethically when we built it, but with the learning of the machines and the models, the outcomes may drift from the expectations. We carefully build in checkpoints to monitor the ongoing ethical issues with potential drift.
Part 4: Applications and use cases
1. What are the applications of artificial intelligence?
a) Real estate
Based on our findings, we believe that the real estate industry is poised for an industry-wide data and AI transformation.
The property industry has lagged other industries in investing and adopting AI but is starting to recognise the value of these capabilities.
AI-generated proptech startups will continue to grow over the coming years, as startups leverage AI to improve an industry starving for innovation.
b) Commercial real estate
Commercial real estate is an ideal candidate for data, insights and AI — primarily due to changing demand for space from tenants.
For example, the co-working trend or working from home through COVID19 will shape the way that people want to work in the future. We can evaluate the data as the market demand shifts and be able to respond to that shift.
For example, Investa has created a product called Fast Lease that allows tenants to sign a simplified, short-term lease and quickly move into the space.
From a data perspective, commercial buildings vary widely in the amount of accessible data that is available. Investa’s newer buildings (e.g. 60 Martin Place), has state-of-the-art sensors and technology that allow us real-time access to building data.
We can use this data in our AI models to develop insights into optimal building operations and preventive maintenance.
Older buildings can be retrofitted with sensors and data can be obtained from siloed systems, but it can be expensive and complicated. Another option is to focus on high-value data sets and prioritise those that will deliver impact.
“Sixty Martin Place is a project that pushed traditional boundaries and challenged the industry by integrating technology into the heart of the development brief, so it was an easy decision for Investa to participate in this endeavour.
Harnessing the combined power of digital twins and augmented reality allows us to provide a previously unexplored user experience through an interactive 3D model of Sixty Martin Place. This ensures owners, tenants and all users who interface with the building can enjoy a superior level of service and interaction with their environment,” said Shen Chiu National Development Director, Investa and Project Director for the Sixty Martin Place development.
“Utilising the open data available in the building, a range of features are currently operational within the AR environment, including a BIM Overlay, IoT data, live temperature sensors and a timeline view of the building. These features offer incredible new management capabilities, specifically across commercial real estate, engineering and insurance industries.”
Investa has also developed a proprietary tenant engagement app called,Insite app, a technology solution that has been invaluable for connecting building occupants with the latest building news, services and events.
The advent of the COVID-19 pandemic saw more people than ever logging onto the app.
“Elevating the way tenants are able to interact with our buildings and with our teams, enables us to deliver a
highly user-centred experience. This has always been central to the way we think about integrating
technology into our buildings and services,” said Sally Franklin, Group Executive for Real Estate Services and Business Operations at Investa.
“We have really seen a dynamic shift in the behaviour of our users. We received amazingly positive feedback from our Digital Wellbeing programs and competitions.
Insite has really bridged the gap between the workplace and the home. Now, we can still connect with our
occupants on a day-to-day basis, we can re-create the workplace virtually and keep that sense of
connectivity alive,” she said.
As the commercial property market transforms, commercial property owners and managers that can use and leverage building data and mine it for insights with AI will have a distinct competitive advantage.
2. Artificial Intelligence as a service (AIaaS)
Following in the footsteps of Infrastructure as a Service (IaaS), Software as a Service (SaaS) and Platform as a Service (PaaS), Artificial Intelligence as a Service (AIaaS) is the third party outsourcing of artificial intelligence.
AI cloud offerings including SparkBeyond, Amazon Machine Learning, Microsoft Cognitive Services and Google Cloud Machine Learning as well as startups like Dataiku, BigML, Forecast. This, can help organisations to understand what might be possible with their data.
AI as a Service allows individuals and companies to experiment with AI for at a fraction of the cost. Before AIaaS, companies had to budget for not only the large initial investment but also the ongoing upkeep of physical and digital equipment. AIaaS allows companies to harness the power of AI at significantly lower costs.
Another benefit of AIaaS is usability. Unlike traditional AI options, which can be open-source and complex, AIaaS provides "plug-and-play" type usability. This allows companies to start utilising AI without needing to employ technical developers and data-scientists upfront.
A final benefit of AIaaS is the ability to scale. As companies become more comfortable with AI, they can expand the use case of AI throughout the organisation. They can also see what works before having to commit to a particular AI solution.
Well-known types of AIaaS include:
● Bots and digital assistance
● Cognitive computing APIs
● Machine learning frameworks
● Fully-managed machine learning services
Unlike other technology, AIaaS is not as easy as "plug and play." The success of AIaaS lies in a strong data capability internally, as well as strong leadership to champion the program.
It also takes significant time and resource investment to really understand and use the software. Those companies that can build this capability have a strong competitive advantage in the property industry. We will continue to see companies outperform that invest in this area.
Through Investa’s partnership with SparkBeyond — an AI/ML software platform — we are achieving strong results, thanks to our strong internal resources and strong leadership support.
3. What is the future of artificial intelligence?
There's no doubt that artificial intelligence is impacting the future of virtually every industry.
AI has also been a primary driver for emerging technologies like big data, robotics and the Internet of Things (IoT). With tech giants like Google, Amazon, Apple and Microsoft, universities and the military, all spending billions of dollars on AI, there is no downturn in sight.
However, there is debate and even concern around exactly how AI will impact life as we know it.
The uncertainty about the future of AI was compounded by billionaire entrepreneur Elon Musk when he said, "AI is a fundamental risk to the existence of human civilisation."
Brad Smith, President of Microsoft said, "Information technology raises issues that go to the heart of fundamental human rights protections, like privacy and freedom of expression. These issues heighten responsibility for tech companies that create these products. In our view, they also call for thoughtful government regulation and for the development of norms around acceptable uses."
The future of AI can be consolidated into four essential parts:
a) The AI-enhanced organisation
AI will continue to provide organisations with tremendous advances in productivity, efficiency, insight, processes and value.
At Investa, building strong capability in the use of AI technologies s is our current pathway and goal. We believe the skills needed to excel in this area can be learnt and we are applying the technology and resulting data visualisation dashboards across a range of business problems, functions and roles.
Organisations that fail to adopt AI and innovate will struggle to survive and compete in the future.
Cognilytica, an AI, Research Advisory and eduction firm believes that AI will enable or augment (rather than replace) work done by humans.
Another predicted future AI trend will be mass-customisation and personalisation at scale. AI will allow companies to deliver a much more personalised, unique experience for customers. This will enhance marketing, sales and customer service functions.
Finally, AI will allow companies to be "always-on" and move beyond standard 9-5 opening hours.
b) Autonomous everything
Autonomous robots, cars, drones and systems are some of the most visible and discussed elements of AI. Autonomous systems can influence retail, health care, journalism and even infrastructure. At Investa, we are currently investigating a range of potential business applications for Drones, which is an exciting opportunity.
“The market and usage of drones is growing quickly, as well as the cost efficiencies they can provide. We’re keenly exploring how we could use drones to add value at Investa, notably in building operations and optimising our space utilisation.” said Anna Bland, Innovation Project Manager.
c) Pervasive knowledge
As AI pushes up the Data-Information-Knowledge-Wisdom (DIKW) Pyramid, society will demand more from governments, companies and each other. People will expect things to be available instantly. Information will need to be accurate and complete and everyone will be connected all of the time.
d) Enhancing the human experience
Using augmented intelligence, AI can enhance and further the creative capabilities of humanity.
AI also can enable humans to communicate with another person in any language and be easily understood — and even address local customs and etiquette.
Conclusion
It is no longer possible to continue with the status quo. Companies that leverage data, insights, AI, ML and automation technologies, will have an increasingly large competitive advantage moving forward.
At Investa, we believe that this technology is the future team member that will handle the complexity of large data sets – allowing us humans to focus on what we do best, being creative and thriving in an environment of ambiguity.
The future is a partnership between humans and machines, where we will be able to deliver better service, better places and better outcomes, as we leverage the power of data, AI and advanced analytics.